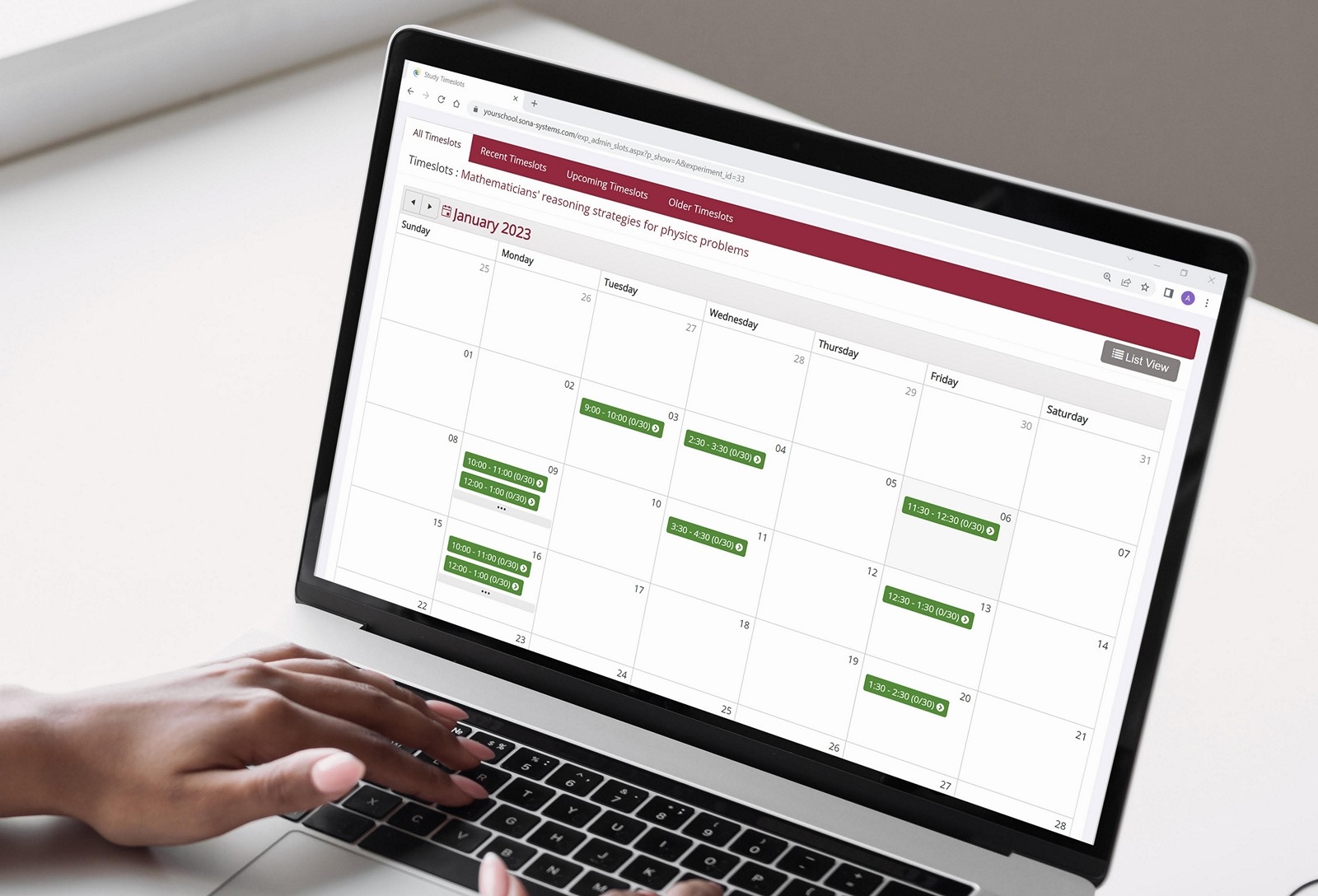
No Shows and Novel Study Designs: Advanced Use of Sona to Advance Science
Published November 3, 2023
Observing the Unobservable: Creativity and Ingenuity in Science
In his 1979 article “Einstein and the Quantum Theory”, Abraham Pais recounts an exchange during one of his many walks (and talks) with Einstein: “I recall that during one walk Einstein suddenly stopped, turned to me and asked whether I really believed that the moon exists only when I look at it.” The question was intended rhetorically. Clearly, the moon is there whether we observe it or not. Yet only three years later, in a paper intended for philosophers of science and physics, physicist N. David Mermin responded rather glibly that “We now know that the moon is demonstrably not there when nobody looks” (see also Mermin’s article “Is the moon there when nobody looks?”). How we can “demonstrably” know anything about what we don’t observe will be an important theme in this post.
Of course, Sona is designed to facilitate research in fields that require participants, not particles. Physics definitely falls into the latter category. That said, from cognitive neuroscience to consumer psychology, fields that fall into the former category are, if anything, even more concerned with measuring the unobservable. Designing studies capable of empirically testing hypotheses about traits, conceptual representation, perceptual processing, learning and memory, and other phenomena not easily or directly observable is a central concern among researchers using Sona. It is one of those aspects of scientific research that too often goes unappreciated. Intuition, creativity, and ingenuity are all essential parts of scientific research that are too often neglected compared to the equally important analytical aspects. This is perhaps nowhere more true than when it comes to translating research questions into study design and protocols.
Consider, for example, the kind of imaginative thought processes that must go into dreaming up a study design able to address a question such as “How do participants who remember X differ from those who don’t?” It’s hard enough to design studies capable of answering the first part of the question, which after all concerns a process that is only indirectly observable. The second part, which concerns an unobservable process that didn’t occur, is more challenging still. But it can be done, it has been done, and Sona can make it easier to accomplish.
To prove it, we want to share with you a brilliantly designed study that used Sona to answer questions about an unobservable process that didn’t occur in participants who weren’t there. If that isn’t creativity at its finest, nothing is.
Sona and Scientific Research
It’s not often that we get to recognize research excellence. This blog is primarily a resource for Sona administrators and researchers rather than a source for research news and reviews. Regardless of the satisfaction and pride we feel whenever we come across good research that used our software, we generally have to leave it at that and let others recognize research excellence. Occasionally, though, we get lucky. Every so often we come across a brilliant study that not only used Sona, it did so in a unique way. We already dedicated a post to such a study, one in which Sona was used in a way that made us jealous we hadn’t thought of it first: Telling Delays: What Students’ Study Sign-up Timing in Sona Reveals.
When a great example of research uses Sona, and does so in a novel and/or unique manner, we get to celebrate it. We have to keep up-to-date with research methods, designs, and tools in many fields. Ingeniously designed studies that use Sona in novel ways constitute a resource we can share, not just something we are proud to be a part of. After all, if we’re impressed by a novel or brilliant use of our software, it’s often because we didn’t see the potential first, and it’s our platform! We’re in a pretty good position to know that others can benefit from hearing about it.
Novel Design in a New Study
Harrington, Reese-Melancon, & Bock’s Prospective Memory Study
Instead of a study on latent learning that would eventually highlight the flaws in the behaviorist paradigm, Harrington et al.’s study concerns prospective memory (PM), which is (in their words) “memory for future events”. More specifically, their study is on one of the two types of PM.
Sona’s Timeslots as Time-Based Prospective Memory
Not surprisingly, Harrington et al. investigated the form of PM less studied of the two (and in many ways the more difficult to study). They note quite early on that, compared to event-based prospective memory (EBPM), time-based prospective memory (TBPM), or “remembering to perform an action at a particular time or after a specified amount of time has passed”, has received comparatively less attention. EBPM “is the remembering what one does when a certain target event occurs. For example, one remembers to buy milk when one passes by the supermarket.” Harrington et al.’s study concerns TBPM, the form of PM less examined and often harder to study.
It’s not difficult to imagine why empirical research on TBPM is rarer than EBPM. Studies can involve future events that, when they occur, are supposed to elicit some measurable response from participants. More complex designs can address events farther in the future (such as with diaries or multi-part studies). But having participants remember to perform some action at a particular future time, and provide valuable data about PM processes at that future time, is more complicated. Doable, but more complicated.
One nice thing about Sona (at least when it comes to TBPM research) is how participants use it to opt into a study and, more importantly, to select a date and time for the study they’re signing up for. Put differently, participants use Sona to schedule a very particular kind of appointment. Part of Harrington et al.’s genius was this realization and the implication: Sona involves time-based prospective memory (well, usually; online studies work differently in terms of scheduling timeslots, but you get the picture).
So far, so good. Timeslots are a kind of appointment that participants have to remember to keep. That certainly qualifies remembering to show up for one’s appointed timeslot a time-based prospective memory. But in order to address important metacognitive aspects of TPBM, Harrington et al. wished to not only examine those who kept their appointments, but those who didn’t.
Harrington et al. had participants complete a survey that, in addition to demographic information, contained two measures relating to prospective memory. One was the Prospective and Retrospective Memory Questionnaire (PRMQ), while the other consisted of questions relating directly to the appointment (i.e., the study) that participants signed up for. All participants signed up to take the survey at an appointed time and place (an in-person timeslot) via Sona. Those who showed up at the appointed time formed the “Show” group. How, though, does one ask the same questions of participants who aren’t there (because they didn’t show)? This is where things really get interesting.
Anybody with any familiarity recruiting participants for studies will be very familiar with the fact that sometimes participants don’t show. This is something researchers typically regard as a negative (if unavoidable) aspect of their work, and so they try to minimize these no-shows. Harrington et al., on the other hand, decided to take this negative and turn it into part of their research design. No-shows are, practically by definition, members of an unobservable population, but Harrington et al. used this participant status in Sona to recruit from an otherwise inaccessible group.
Instead of the typical ways researchers have when it comes to no-shows (allow for additional sign-ups, account for these no-shows statistically, etc.) the researchers regarded them as a sample from a population of considerable interest:
“The No-Show sample consisted of participants who signed-up to attend an in-person research session between September 2018 and March 2020 but failed to attend…When a student registered for the in-person research session, they chose a time to participate, and the research system documented the student’s name, email address, and appointment time. When the student failed to attend their appointment at the designated time, a researcher (i.e., the first author) sent the student an email inviting them to participate in an online survey that needed to be completed within 24-hours.”
From Protocols to Practical Matters
Much of the novelty and ingenuity in Harrington et al’s use of Sona is how they were able to recruit participants based on non-standard criteria. They were able to quickly reach out to participants who signed up for a study in order to invite them into another study even though they had no way of knowing a priori which participants would “qualify” for the second study.
In our existing resources, we go over in some detail the kinds of ways to implement both online and in-person multi-part studies, invite qualified participants, and other aspects that are similar to the methodology employed by Harrington et al. As we’ve said several times already, though, their recruitment strategy, at least for the No Show group, was particularly creative. Better still, it’s the kind of design that can be generalized for similar uses in quite different contexts. This makes it more than worthwhile to take a small detour into how any researcher can implement the procedure that Harrington et al. employed.
This is particularly true because there isn’t just one way to identify no-shows (or other participant statuses conditional upon a particular study and/or timeslot). Sona provides researchers multiple ways to quickly contact no-shows (and participants meeting other criteria) from a given study or even a particular timeslot. Perhaps the simplest is to select “Contact Participants” from the study menu options and select the option for “No-Shows”:
Another method would be to simply track status changes in timeslots and contact participants using the email feature on this page:
And there’s no reason to employ only one strategy as long as it is clearly defined at the outset. For example, although we generally recommend, for lab-based/in-person studies, that researchers mark participation as soon as the timeslot has ended, in cases like this the first step could be to contact no-shows, then to mark participation. Also keep in mind what data researchers on your Sona site will have access to and what details participants have provided. In some cases, the participant list for a given study will contain all their email addresses, in others this might not be so.
Whatever the method employed, Sona makes it easy to consider no-shows as members of an otherwise largely inaccessible population. No-show status, however, is by no means the only useful criterion when it comes to creative study designs.
Lessons Learned and Key Takeaways
Before we get into the core takeaways, we should lay a little more groundwork here. Remember that the goal here isn’t simply to recognize great science and a brilliantly conducted study. It’s to use these methods to further scientific research more generally. This means examining not the study details but the methodological structure (the design) of the study. It means abstracting away from the specific context to allow other researchers working in different contexts to make use of, even extent, the methods employed. For scientists, most of the time the work is all about getting from the abstract to the realization and implementation of a particular study’s details in the context of a specific research question. As a research platform, however, Sona is of necessity and by design a tool flexible enough to accommodate not only different contexts, but entirely different sciences. We are able to ensure this in part because we frequently must analyze research processes in reverse. That is, we tend to look at many different research contexts and study particulars and abstract from the specifics to find the more general. We’re going to give you an inside glance at this process using Harrington et al.’s study as an example.
Sona and the Structure of Studies
We want to look at the study by Harrington et al. with an eye to generalizing their use of Sona to future research. Clearly, simply noting that Harrington et al.’s study had two parts is too abstract. But it’s an interesting starting place. Whenever you add a study in Sona, you are given the option to select a multi-part study. However, such studies (with or without Sona) are aimed at getting all participants to take part in all parts of the study. Often, though, this isn’t the most important consideration. Indeed, as Harrington et al.’s example show, sometimes it isn’t even desirable.
We can easily imagine scenarios in which researchers would wish to invite only some participants from a standard lab study. Imagine a research group studying math anxiety and mathematics education. Perhaps they are interested in determining the optimal conditions for students with math anxiety to take mathematics exams. Having a group of students take a math test is pretty easy, as it tends to happen every semester. But if researchers are then interested in selecting subsets of these and inviting them to participate in a study that involves taking another, similar exam under different conditions, this can be trickier. Despite the vastly different research context, though, the method Harrington et al. use can be employed here as well. Students who scored high on measures of math anxiety and performed poorly could be invited to take a similar exam online to compare scores from a test taken in the classroom to those from a test taken in a comfortable, self-selected environment.
In general, the kind of study protocols and research designs that we get by looking abstractly at the structure of Harrington et al.’s work is something we might call conditional multi-part studies. That is, a study that is designed to have two or more components, but who qualifies as a participant in later parts is conditional upon earlier. To simplify, we’ll consider just the two-part case and call the parts of the study Study 1 and Study 2.
There are many scenarios in which researchers know ahead of time that they’ll want to invite some participants from Study 1 to take part in a follow-up study. It could be that some participants from Study 1 are invited to take part in Study 2 based on meeting certain conditional criteria. But it could equally be something more complex, in that perhaps all participants will be invited to take part in one of several different follow-up studies depending upon information obtained from Study 1. To use the math anxiety example again, perhaps there are several different testing conditions and environments, e.g., online, in a smaller classroom, in a room alone, in a large lecture hall, outside in a group, etc., that researchers wish to have participants take a mathematics exam and which partipants qualify as belonging to which groups depends up on both their performance on a math test in Study 1 as well as a questionnaire they filled out after completing their math exam.
Granted, this is all still pretty abstract even with an example to have in mind, but we hope you can get a sense of just how vast the range of applications here is. There are many research questions that can be better addressed when researchers can more easily recruit some participants from one study to take part in a follow-up study, or one out of several follow-up studies. Sometimes this is possible to do using any of the multiple built-in methods Sona has for setting study eligibility. Often, however, the follow-up might be time sensitive enough that these aren’t optimal. Something more like the method Harrington et al. used, though, may very well be.
Experimental Methods, Measures & Designs with Sona
We want to end with an eye to the future. Not just future studies and their design, but the role Sona plays in the design and implementation of research. A large part of Sona’s role is in structuring (even standardizing) the research process. It is only natural, then, that we tend to look at this process in the most general terms and at the most abstract levels first, and then proceed to specifics. Even then, however, as a software platform that must be used in numerous contexts by various researchers across the sciences, our concern with specific research contexts is ensuring our platform remains the optimal manner in which to address these (providing, of course, the context involves a participant pool).
Study protocols, research methodology, and experimental designs must, by their very nature, be generalizations that abstract away from any specific realization or implementation. Harrington et al. did great work to be sure, and their study is a itself a study in ingenuity (pardon the pun). For it to be a building block for future studies, though, requires looking also at their study through the abstract lens we discussed above, and attempting to capture essential structures from their research process that others can use.
Inevitably, such abstractions come at a cost. We cannot generalize without losing some of those aspects of the study by Harrington et al. that are too specific to a particular research context. To make matters worse, perhaps the most brilliant aspect of Harrington et al.’s work and of their use of Sona falls precisely into this category. They were interested in prospective memory. They needed a task participants could perform that would constitute the use of prospective memory.
This is what allowed them to use Sona as their PM task: Sona enables participants to sign-up for timeslots, timeslots are appointments, ergo Sona study sign-ups are a PM task. Better yet, those who did not show up for the appointment automatically became an otherwise inaccessible population that they were again able to access and compare to the Show group via Sona. Alas, this is a very specific sort of task and not among the aspects of their study that can be readily generalized.
What can be generalized is their implementation of a conditional multi-part study. We discussed this already in the previous section. Our focus here is different. Our efforts to make our platform seamlessly integrate with the research process have been so effective that researchers can almost forget how large a role Sona plays. This is not necessarily a bad thing. We are, after all, trying to make the lives of researchers, participants, instructors, and administrators much easier. It can be a negative, however, if researchers come to think of Sona as nothing more than a background process.
The study by Harrington et al. shows why such a view is mistaken and how valuable it can be to better understand Sona’s capabilities. Whether or not participants show up for a study need not be a purely administrative question, as shown by this study. Many other aspects of our platform can serve a variables, measures, tasks, and more in a wide variety of contexts. A great many scientists in different fields are interested in the role of time in everything from clinical contexts to cognitive processes. Sona makes it easy to ensure that all timeslots fall into either an “early” or “late” time. Location too may be a variable. In many circumstances, the prescreen can serve in place of the information that an initial study would provide, allowing researchers to begin “Study 1” as a set of possible different study conditions that use prescreen restrictions to determine which groups of participants are invited to take part condition.
None of these ways of using Sona’s tools, however, can match the versatility of conditioning recruitment for future or follow-up studies on some information gained from a previous study. It may be oversimplifying somewhat, but essentially all multi-part studies with human participants either involve recruiting participants for each part, using methods such as the built-in tools Sona offers, or inviting participants to take part in future studies based on conditions that will be known from earlier ones. The possibilities are endless, and the limits are as boundless as one’s scientific creativity.
Concluding Thoughts
That, in a nutshell, is the real takeaway from Harrington et al.’s study on prospective memory. To be sure, it’s not the key takeaway of the study. Harrington et al.’s outstanding research has much to offer memory research and contains much we couldn’t cover, no matter how great we think it is. For us, at least in this particular context (i.e., here at Sona, on the Sona blog, writing to an audience about the Sona platform), the key takeaway from their study and this discussion is the example they set from a methodological perspective. By creatively using Sona to recruit participants based on conditional information from an initial study (and, recall that in this case, the information was merely that participants didn’t show!), we have a very clean, illustrative instance of how to conduct a conditional multi-part study.
By structuring the research process, Sona becomes integral to the research process and a valuable research tool in ways that even we can’t predict. That’s a lesson we learned from Harrington et al. and one definitely worth sharing.